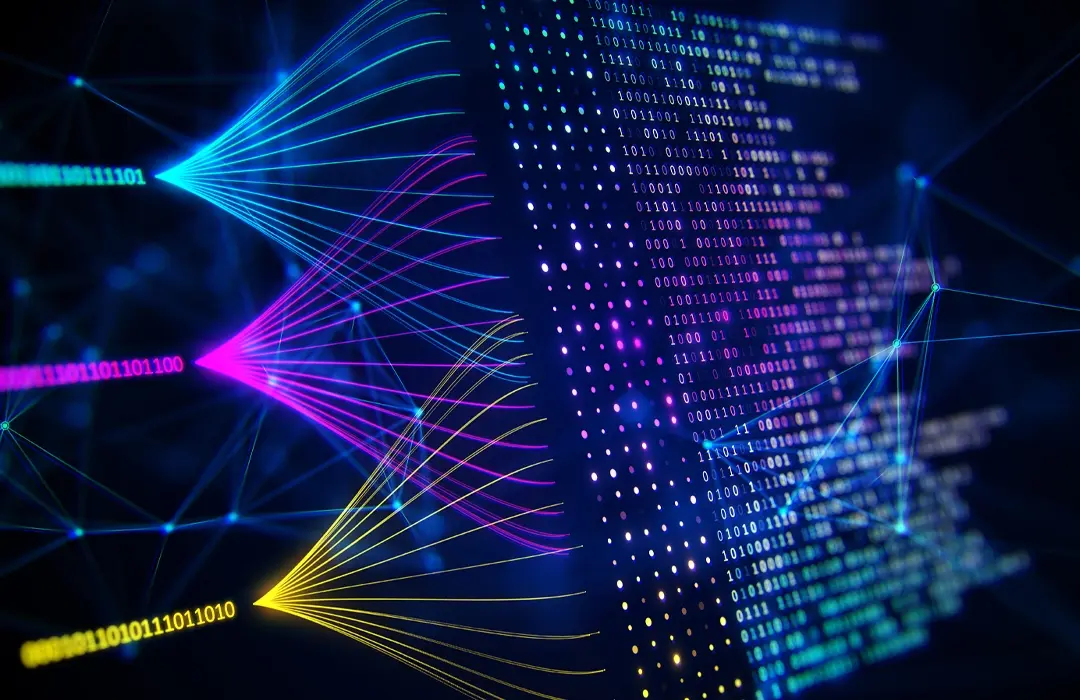
$20 GPT-4 Subscription or Amazon Bedrock: Which One Should Power Your In-House Tool?
People say “AI for this, AI for that,” yet most people use AI like grown-ups using their smartphones—they barely scratch the surface. But you’re not most people. You’re an LLM expert, or maybe part of an insurance claims adjustment team, considering purchasing a paid version of OpenAI’s GPT-4.0 to get faster and more accurate results. Your team deals with thousands of claims every day, which means:
- Reading through lengthy documents to identify relevant policy terms
- Match them with incident reports
- Flag potential frauds
It’s slow, manual, and draining. So, why not bring in GPT-4 and speed things up? Seems like a smart move—until you realize GPT-4 doesn’t offer the kind of enterprise-grade data protection your business needs. What if the model starts learning from your data? What if your internal claims logic gets baked into the model and ends up helping a competitor? You’re not just buying a faster tool—you could be risking your own IP.
That’s a real-world problem, and this is exactly where Amazon Bedrock steps in. It lets you build your in-house AI tools using the same powerful foundational models, without your data ever leaving your control.
What is Amazon Bedrock?
Let’s say you’re a tech lead, a CTO, or even a CIO. Knowing about Amazon Bedrock probably isn’t high on your priority list—you’ve got bigger things to worry about, like running day-to-day operations. Maybe you’re thinking, “My team will handle all this.” But here’s the thing: you’re wrong. The implementation of foundational tools like Bedrock starts at the top. If leadership isn’t invested in how AI is used internally, especially in mission-critical, data-sensitive workflows, then why should your team be? Culture, compliance, and capability all begin with you.
Think of Amazon Bedrock as a secure AI playground where you get access to the best foundational models, like those from Anthropic (Claude), AI21 Labs, Meta (LLaMa), and even Stability AI. But here’s the kicker: you don’t have to build or train anything from scratch. You can plug these models directly into your systems and workflows—no servers, no infra headaches, no IP leakage.
Let us take the previous example where you are leading a team of an insurance claims adjuster team. Using Amazon Bedrock, you can create an automation tool that can read documents, flag inconsistencies, and summarize what you need to do. Now, what do you need next? You need to train the model with internal data, and that too securely, making sure that it can be done too, using this. With Bedrock, you bring in the logic and the data, while Amazon brings in the horsepower.
The diagram above demonstrates the architecture and key components of a claims automation tool built and scaled with Amazon Bedrock.
GPT-4 Subscription vs. Bedrock: What Are You Really Buying?
At first glance, $20 a month for GPT-4 sounds like a steal. You get access to one of the world’s most advanced language models, faster responses, and better reasoning. For individual use or small experiments, it’s unbeatable. But once you move into enterprise territory—where customer data, internal logic, and compliance are non-negotiables—the math starts to change.
Let’s break it down.
With a GPT-4 subscription:
- Your prompts and data go to OpenAI’s servers.
- You can’t fully control how the model uses or retains that data.
- There’s no simple way to integrate it into your infrastructure.
- You’re relying on a consumer-facing product to power enterprise workflows.
It’s like using a kitchen knife to cut wood—technically possible, but far from ideal.
With Amazon Bedrock:
- Your data stays within your AWS environment—no exposure to public APIs.
- You can plug foundational models into your systems, securely and at scale.
- You decide what data the model sees, remembers, and acts on.
- You can access multiple leading models (Anthropic’s Claude, Meta’s LLaMa, etc.)—not just one.
Most importantly, you’re not just buying speed. You’re buying control over your data, your workflows, and the future of your organization’s use of AI.
So ask yourself:
Do you want to play with AI? Or do you want to build with it? So, what does it look like when you have actually deployed it in a real-life project?
How it looks in Practice: Scaling Securely with Bedrock
Let us go back to our previous example of an insurance claims adjuster. What they required was a basic automation tool that reads claim forms, checks them against policy documents, and flags inconsistencies.
That alone saves your team hours every week. But the real magic happens when you scale this securely.
Here’s what that looks like:
- You train the model on historical claims data within your secure AWS environment. No data leaves your infrastructure. You control what’s shared and what stays locked down.
- You integrate it with your internal systems. That means your claims processing tool isn’t a separate silo—it plugs into your existing CRMs, document repositories, and fraud detection systems.
- You fine-tune workflows. Over time, your team stops reviewing low-risk claims manually. The tool triages them automatically, escalating only the ones that truly need human oversight.
- You audit everything. Because it’s running in your own AWS space, every model decision is traceable. You stay compliant, and your risk and legal teams stay happy.
Today, something new drops every other day. AI has gone from “wow” to wallpaper. ChatGPT is writing homework, Ghibli-style AI images are flooding the internet, and everyone’s shouting “AI-first!” But here’s the thing— it’s no longer about using AI for smarter workflows. It’s about choosing an infrastructure you can trust.
Amazon Bedrock doesn’t just give you access to powerful models.
It gives you control over your data, your workflows, and your competitive edge.
You’re not just moving faster. You’re building systems that learn, adapt, and grow securely.
At the end of the day, it’s not about choosing the “best” tool.
It’s about choosing the one that works best for you.